A Comprehensive Guide to MOAs: Practical Examples in Adaptive Computation and Machine Learning

Meta-Optimizers (MOAs) have emerged as a powerful tool in adaptive computation and machine learning, enabling the optimization of algorithms and models by learning from past experiences. This comprehensive article provides a deep dive into MOAs, exploring their significance, benefits, challenges, and practical applications in various domains. Through real-world examples, we will demonstrate the effectiveness of MOAs in enhancing the performance of machine learning algorithms and models.
4.1 out of 5
Language | : | English |
File size | : | 20296 KB |
Text-to-Speech | : | Enabled |
Screen Reader | : | Supported |
Enhanced typesetting | : | Enabled |
Print length | : | 355 pages |
Paperback | : | 194 pages |
Item Weight | : | 10.2 ounces |
Dimensions | : | 6 x 0.44 x 9 inches |
Understanding Meta-Optimizers (MOAs)
MOAs are higher-level optimizers that operate on a meta-level, controlling the learning process of other algorithms or models. They learn from past optimization experiences and adapt the learning process to improve the performance of the underlying algorithms. By adjusting hyperparameters, neural architectures, or even the optimization algorithms themselves, MOAs enhance the efficiency and effectiveness of machine learning systems.
The key advantage of MOAs lies in their ability to automate the optimization process, reducing the need for manual tuning and experimentation. This not only saves time and resources but also enables the exploration of a wider range of optimization options, leading to improved performance.
Practical Examples of MOAs
- Hyperparameter Optimization: Hyperparameters are parameters that control the learning process of machine learning algorithms. MOAs can optimize these hyperparameters to enhance the performance of the algorithm. For instance, in neural networks, MOAs can optimize the learning rate, batch size, and regularization parameters.
- Neural Architecture Search: MOAs play a crucial role in Neural Architecture Search (NAS),where they search for optimal neural network architectures. By combining evolutionary algorithms with reinforcement learning, MOAs can generate and evaluate candidate architectures, leading to the design of more efficient and accurate neural networks.
- Evolutionary Algorithms: MOAs can be used to optimize the parameters and operators of evolutionary algorithms, enhancing their search capabilities. In genetic algorithms, for example, MOAs can adjust the mutation rate and crossover probability, improving the exploration and exploitation balance.
- Bayesian Optimization: MOAs can guide the acquisition function in Bayesian optimization, a technique for optimizing black-box functions. By learning from past observations, MOAs can suggest promising regions for exploration, accelerating the optimization process.
Benefits and Challenges of MOAs
Benefits:
- Automated optimization process
- Improved performance of machine learning algorithms and models
- Reduced time and resources for optimization
- Exploration of a wider range of optimization options
Challenges:
- Computational cost of optimization
- Overfitting to specific tasks or datasets
- Limited interpretability of optimization decisions
Future Research Directions
The field of MOAs is rapidly evolving, with several exciting research directions emerging:
- Scalable MOAs: Developing MOAs that can handle large-scale optimization problems with complex models
- Transferable MOAs: Exploring MOAs that can transfer knowledge and strategies across different tasks and domains
- Interpretable MOAs: Enhancing the interpretability of MOAs to gain insights into their optimization decisions
Meta-Optimizers (MOAs) have revolutionized adaptive computation and machine learning, providing a powerful tool for optimizing algorithms and models. Through practical examples, we have demonstrated the effectiveness of MOAs in enhancing the performance of machine learning systems. As research continues to advance, we can expect even more innovative and sophisticated MOAs that will further push the boundaries of machine learning.
4.1 out of 5
Language | : | English |
File size | : | 20296 KB |
Text-to-Speech | : | Enabled |
Screen Reader | : | Supported |
Enhanced typesetting | : | Enabled |
Print length | : | 355 pages |
Paperback | : | 194 pages |
Item Weight | : | 10.2 ounces |
Dimensions | : | 6 x 0.44 x 9 inches |
Do you want to contribute by writing guest posts on this blog?
Please contact us and send us a resume of previous articles that you have written.
Chapter
Text
Story
Genre
Reader
Library
Magazine
Paragraph
Sentence
Bookmark
Shelf
Glossary
Bibliography
Foreword
Preface
Synopsis
Annotation
Footnote
Manuscript
Tome
Library card
Autobiography
Memoir
Encyclopedia
Dictionary
Resolution
Card Catalog
Borrowing
Stacks
Archives
Research
Lending
Academic
Special Collections
Literacy
Thesis
Dissertation
Storytelling
Reading List
Textbooks
Michelle Celmer
E Ayca Roel
Marcus Gray
Philip Wu
Carol Belanger Grafton
Marcy Tilton
C Alexander London
Michael Ezeanaka
Claudia Burgoa
Jamie Brenner
W James Dennis
Krista Ritchie
Bruce Catton
Carla Damron
Jerrold Lerman
Joe Parkinson
Christopher Duval
Juliana Sissons
Rug Hooking Magazine
Wallace Wang
Light bulbAdvertise smarter! Our strategic ad space ensures maximum exposure. Reserve your spot today!
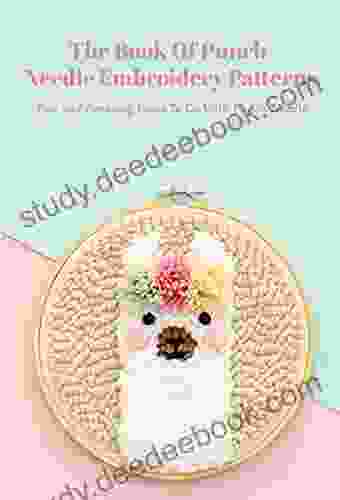

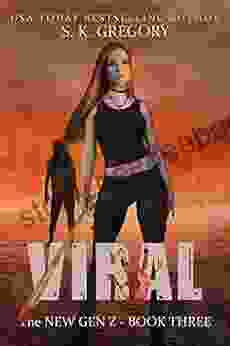

- Oliver FosterFollow ·10.4k
- Harvey HughesFollow ·5.4k
- Damon HayesFollow ·9.6k
- Hunter MitchellFollow ·18.1k
- Boris PasternakFollow ·16.3k
- Russell MitchellFollow ·9.6k
- Tyrone PowellFollow ·9.1k
- Jeffery BellFollow ·17.9k
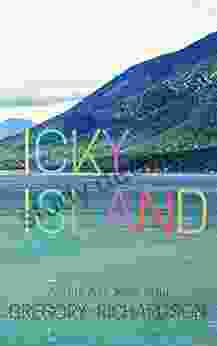

Icky Island: An Unforgettable Adventure for Kids!
Introducing Icky Island: A Delightful One...
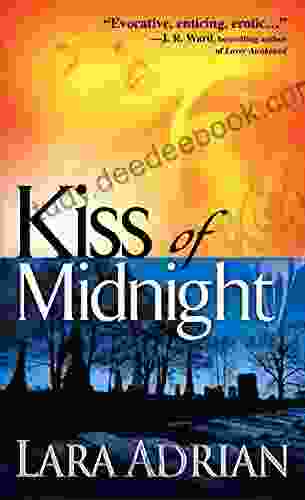

The Midnight Breed: Embracing the Shadows and Unlocking a...
Welcome to the captivating world of...
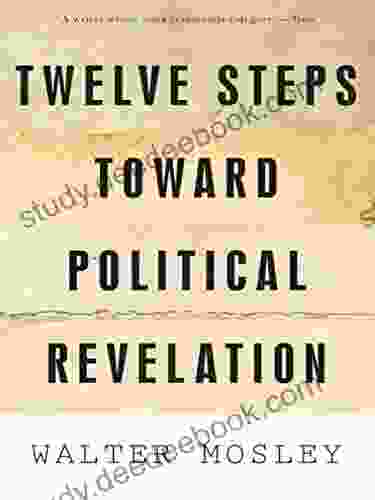

Twelve Steps Toward Political Revelation: A Path to...
Politics, often perceived as a complex and...
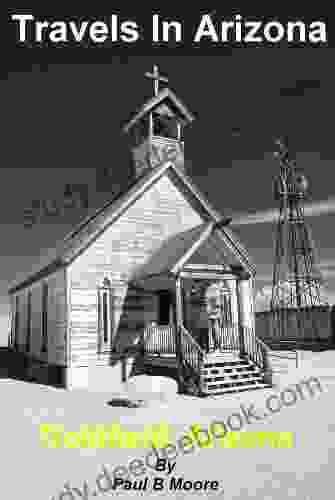

Travels in Arizona Goldfield: Unraveling the Threads of...
Nestled amidst the rugged...
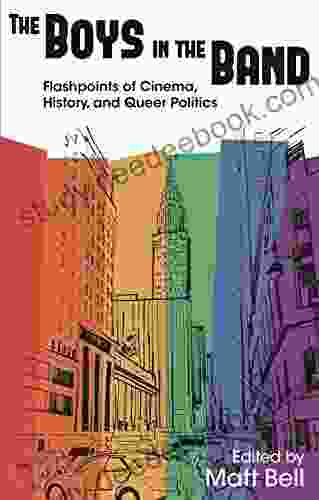

Flashpoints of Cinema History and Queer Politics:...
The relationship between cinema history and...
4.1 out of 5
Language | : | English |
File size | : | 20296 KB |
Text-to-Speech | : | Enabled |
Screen Reader | : | Supported |
Enhanced typesetting | : | Enabled |
Print length | : | 355 pages |
Paperback | : | 194 pages |
Item Weight | : | 10.2 ounces |
Dimensions | : | 6 x 0.44 x 9 inches |