Tackling Data Analytics and Machine Learning Challenges: A Comprehensive Guide to Building Complex Solutions

5 out of 5
Language | : | English |
File size | : | 29850 KB |
Text-to-Speech | : | Enabled |
Screen Reader | : | Supported |
Enhanced typesetting | : | Enabled |
Print length | : | 666 pages |
In the era of digital transformation, data analytics and machine learning (ML) have emerged as critical tools for businesses seeking to gain insights, predict outcomes, and make data-driven decisions. However, these technologies come with their own set of challenges that can hinder their effective implementation. This comprehensive guide will delve into the complexities of data analytics and ML, exploring the common challenges and providing practical strategies to overcome them. By addressing these hurdles, organizations can unlock the full potential of data and build complex solutions that deliver tangible business outcomes.
Understanding the Challenges
The realm of data analytics and ML presents several hurdles that can impede progress. These challenges include:
1. Data Quality and Accessibility:
Poor data quality, inconsistencies, and limited accessibility can hinder the accuracy and reliability of data analytics and ML models. Organizations must establish robust data governance practices to ensure data integrity and seamless access.
2. Model Complexity and Interpretability:
Complex ML models often require specialized expertise to build and interpret, making it challenging for non-technical stakeholders to comprehend and trust the insights derived from them. Striking a balance between model complexity and interpretability is crucial.
3. Data Volume and Scalability:
Big data environments pose challenges in terms of storage, processing, and analysis. Organizations need scalable solutions to handle massive datasets and extract meaningful insights efficiently.
4. Lack of Skilled Resources:
The demand for data scientists and ML engineers far exceeds the supply, creating a talent shortage that can slow down project implementation. Investing in upskilling and training initiatives is essential to address this gap.
5. Ethical and Regulatory Concerns:
Data privacy, bias, and the ethical implications of ML algorithms raise concerns that need to be carefully addressed. Organizations must comply with relevant regulations and establish ethical frameworks to guide their data analytics and ML practices.
Overcoming the Challenges: A Step-by-Step Guide
To overcome these challenges and harness the transformative power of data analytics and ML, organizations can adopt the following strategies:
1. Data Preparation and Governance:
Prioritize data quality by implementing data cleansing, standardization, and validation processes. Establish clear data governance policies and roles to ensure data integrity and accessibility.
2. Iterative Model Development and Validation:
Start with simple and interpretable models, and gradually increase complexity as needed. Validate models thoroughly using cross-validation and real-world data to ensure accuracy and reliability.
3. Scalable Infrastructure and Algorithms:
Choose cloud-based platforms or distributed computing architectures to handle big data volumes. Explore scalable ML algorithms, such as distributed gradient boosting, to train models efficiently.
4. Upskilling and Collaboration:
Invest in training programs to bridge the skills gap. Encourage cross-functional collaboration between data scientists, engineers, and business stakeholders to foster a shared understanding of data analytics and ML concepts.
5. Ethical and Regulatory Compliance:
Establish a framework for responsible data handling, bias mitigation, and algorithmic transparency. Comply with relevant data protection regulations and seek guidance from experts in the field.
Building Complex Solutions
By overcoming the aforementioned challenges, organizations can embark on the journey of building complex data analytics and ML solutions that address real-world business problems. These solutions involve:
1. Predictive Analytics:
Leverage ML algorithms to build predictive models that forecast future outcomes and identify trends. This enables informed decision-making and risk mitigation.
2. Recommender Systems:
Develop personalized recommendations based on user behavior and preferences. Enhance customer engagement and drive business growth.
3. Anomaly Detection:
Detect deviations from normal patterns in data to identify potential problems or opportunities. Improve system performance and enhance security.
4. Image and Speech Recognition:
Utilize deep learning techniques to recognize objects, interpret speech patterns, and make data more accessible. Unlock new possibilities in fields such as healthcare, retail, and entertainment.
5. Natural Language Processing:
Analyze and extract insights from unstructured text data, such as social media posts or customer feedback. Improve customer understanding and enhance decision-making.
Tackling the challenges of data analytics and ML is a crucial step towards unlocking the transformative potential of these technologies. By understanding the complexities, adopting practical strategies, and building complex solutions, organizations can gain data-driven insights, optimize operations, and drive innovation. This comprehensive guide provides a roadmap for organizations to overcome these hurdles and embrace a data-centric future.
5 out of 5
Language | : | English |
File size | : | 29850 KB |
Text-to-Speech | : | Enabled |
Screen Reader | : | Supported |
Enhanced typesetting | : | Enabled |
Print length | : | 666 pages |
Do you want to contribute by writing guest posts on this blog?
Please contact us and send us a resume of previous articles that you have written.
Novel
Page
Chapter
Text
Reader
Paperback
Magazine
Shelf
Glossary
Bibliography
Foreword
Annotation
Footnote
Scroll
Tome
Classics
Library card
Narrative
Biography
Autobiography
Encyclopedia
Dictionary
Thesaurus
Character
Resolution
Librarian
Card Catalog
Borrowing
Stacks
Scholarly
Lending
Rare Books
Interlibrary
Study Group
Thesis
Awards
Reading List
Book Club
Theory
Textbooks
Tamara Draut
Thomas Otway
Nancy Marie Mithlo
Martina Boone
Bookdrawer
Stephen Mitchell
Diane Andrews Henningfeld
Linda Polman
Neil Hanson
Marilyn Bogusch Pryle
Buddhist Text Translation Society
Anosh Irani
C K Prahalad
Toby Neighbors
Jacqueline Pearce
Rita Mae Brown
Phil Kaye
Michael Chase
Gemma Rogers
Christian Davenport
Light bulbAdvertise smarter! Our strategic ad space ensures maximum exposure. Reserve your spot today!
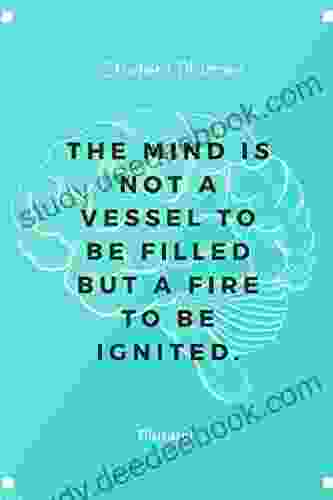

- Devin RossFollow ·13.8k
- Vernon BlairFollow ·15.8k
- Mike HayesFollow ·15k
- Douglas FosterFollow ·18.5k
- Jedidiah HayesFollow ·7.3k
- Steve CarterFollow ·10.4k
- Arthur Conan DoyleFollow ·15.8k
- Doug PriceFollow ·18.1k
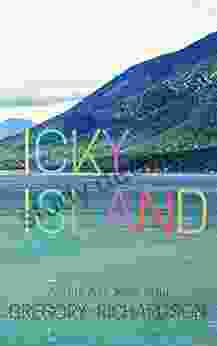

Icky Island: An Unforgettable Adventure for Kids!
Introducing Icky Island: A Delightful One...
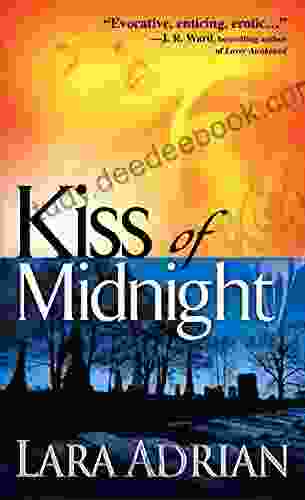

The Midnight Breed: Embracing the Shadows and Unlocking a...
Welcome to the captivating world of...
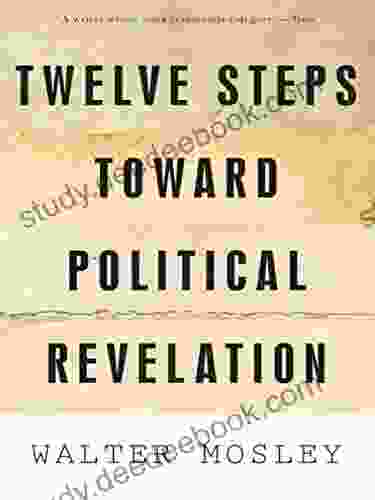

Twelve Steps Toward Political Revelation: A Path to...
Politics, often perceived as a complex and...
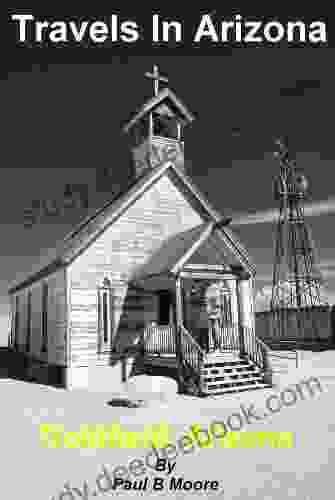

Travels in Arizona Goldfield: Unraveling the Threads of...
Nestled amidst the rugged...
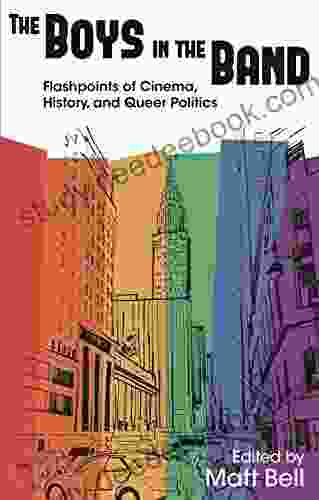

Flashpoints of Cinema History and Queer Politics:...
The relationship between cinema history and...
5 out of 5
Language | : | English |
File size | : | 29850 KB |
Text-to-Speech | : | Enabled |
Screen Reader | : | Supported |
Enhanced typesetting | : | Enabled |
Print length | : | 666 pages |